
Table of Contents
Revolutionizing AI in Healthcare: CHAI Introduces Open-Source Nutrition Label Model Card
CHAI introduces an open-source "Nutrition Label" Model Card for healthcare AI, promoting transparency, trust, and collaboration to improve patient care.
Revolutionizing AI in Healthcare: CHAI Introduces Open-Source Nutrition Label Model Card
The world of healthcare is rapidly evolving, and artificial intelligence (AI) is at the heart of this transformation. A recent initiative by the Coalition for Health AI (CHAI) introduces the concept of a "Nutrition Label" Model Card for healthcare AI systems. According to Healthcare IT News, the goal behind this open-source solution is to foster transparency, trust, and accessibility in an industry that deals with life-altering decisions. But what are the implications of this innovation, and how might it reshape healthcare as we know it?
The Nutrition Label for AI: A New Standard for Transparency
The idea of a "Nutrition Label" for AI might sound unconventional, but its purpose is well-founded. Much like food nutrition labels help consumers understand what they're consuming, CHAI's model card aims to provide users with essential information about AI tools and systems. From performance metrics to data source details, the card intends to offer a comprehensive look at what’s under the hood of these powerful technologies.
Why is this necessary? The healthcare sector has increasingly come to rely on AI for tasks ranging from diagnostics to workflow management. However, this reliance comes with risks, including bias, inaccuracy, and potential misuse of AI systems. The introduction of an open-source, standardized framework could drastically improve clinicians' and patients' confidence in these tools. As quoted in the article, CHAI Director Dr. Brian Anderson notes, "Transparency is paramount for fostering trust in AI systems that impact people’s health outcomes."
Breaking Down the Model Card
CHAI’s Nutrition Label Model Card is designed to address some of the shortcomings in existing AI systems. Key areas of focus include:
- Data Provenance: Where and how was the data used to train the AI collected?
- Performance Indicators: How accurate is the AI, and under what conditions does it perform well—or poorly?
- Bias Mitigation: What steps have been taken to minimize biases, and what residual biases may remain?
- Intended Use Cases: What are the approved applications of this AI tool?
By standardizing these elements into a single, easy-to-understand framework, the Nutrition Label Model Card could help both developers and users align on the capabilities and limitations of their tools. Moreover, as it is open-source, this allows for an industry-wide collaboration aimed at refining and improving the model over time.
Why Open Source? The Power of Collaboration
A significant aspect of this initiative is its open-source nature. By making the Nutrition Label Model Card framework freely accessible, CHAI is encouraging widespread adoption and collaborative improvement. Developers worldwide can adapt the framework to their specific tools or add new metrics to improve its robustness.
Though some proprietary AI vendors might be skeptical of open-source transparency, CHAI’s decision aligns with broader trends in tech and healthcare. Open standards often lead to better innovation as they lower the barriers to entry while enhancing accountability. For healthcare AI—an industry balancing innovation with strict ethical demands—this could be a game-changer.
Potential Challenges and Questions for the Future
While the Nutrition Label Model Card appears to be a giant leap forward, it raises several important questions:
- Adoption Rates: Will major AI vendors be willing to adopt this open-source standard, particularly those operating with proprietary or opaque systems?
- Interpretability: The model card aims to be user-friendly, but will it truly be accessible to non-experts, such as patients themselves?
- Enforcement: Without regulatory mandates, how will the adoption of these model cards be tracked and enforced across the industry?
- Bias and Limitations: While the framework focuses on illuminating biases, it does not eliminate them. Can further innovation ensure these limitations are minimized?
These questions underscore the challenges the healthcare ecosystem might face in fully integrating transparent AI practices. Nevertheless, these challenges may serve as opportunities for sharpening the model card’s focus and advocating for regulations that support its adoption.
The Balancing Act: Optimism Met with Skepticism
The launch of the Nutrition Label Model Card demonstrates CHAI’s commitment to ethical AI development, but it’s important to temper optimism with measured skepticism. On one hand, these cards represent a necessary step toward making AI tools safer and more effective. On the other hand, the initiative relies heavily on voluntary participation and self-regulation—a strategy that hasn’t always proven effective in other industries.
Additionally, while the model card focuses on transparency, transparency alone does not guarantee improvement. For example, revealing that a particular AI tool performs poorly on minority populations raises awareness but does not solve the core issue. Addressing these gaps would require a deeper systematic overhaul, which cannot be achieved by transparency initiatives alone.
Moreover, global healthcare systems vary widely, and the one-size-fits-all nature of an open-source framework may face hurdles in adapting to localized needs. It will be intriguing to watch how developers in varied healthcare settings implement and tailor these model cards.
Final Thoughts
CHAI’s Nutrition Label Model Card represents an important milestone in the journey toward ethical, transparent healthcare AI. With the rise of tools that influence critical medical decisions, fostering trust and accountability is no longer optional—it’s imperative.
As this initiative unfolds, it will provide a crucial case study for the role of open-source, standardized frameworks in shaping the future of healthcare AI. Whether it sets a new benchmark or faces resistance, only time will tell. What remains clear is that innovations like this are essential to achieving the delicate balance between AI’s immense potential and the safety of the human lives it impacts.
For more details about this groundbreaking initiative, you can read the original article on Healthcare IT News.
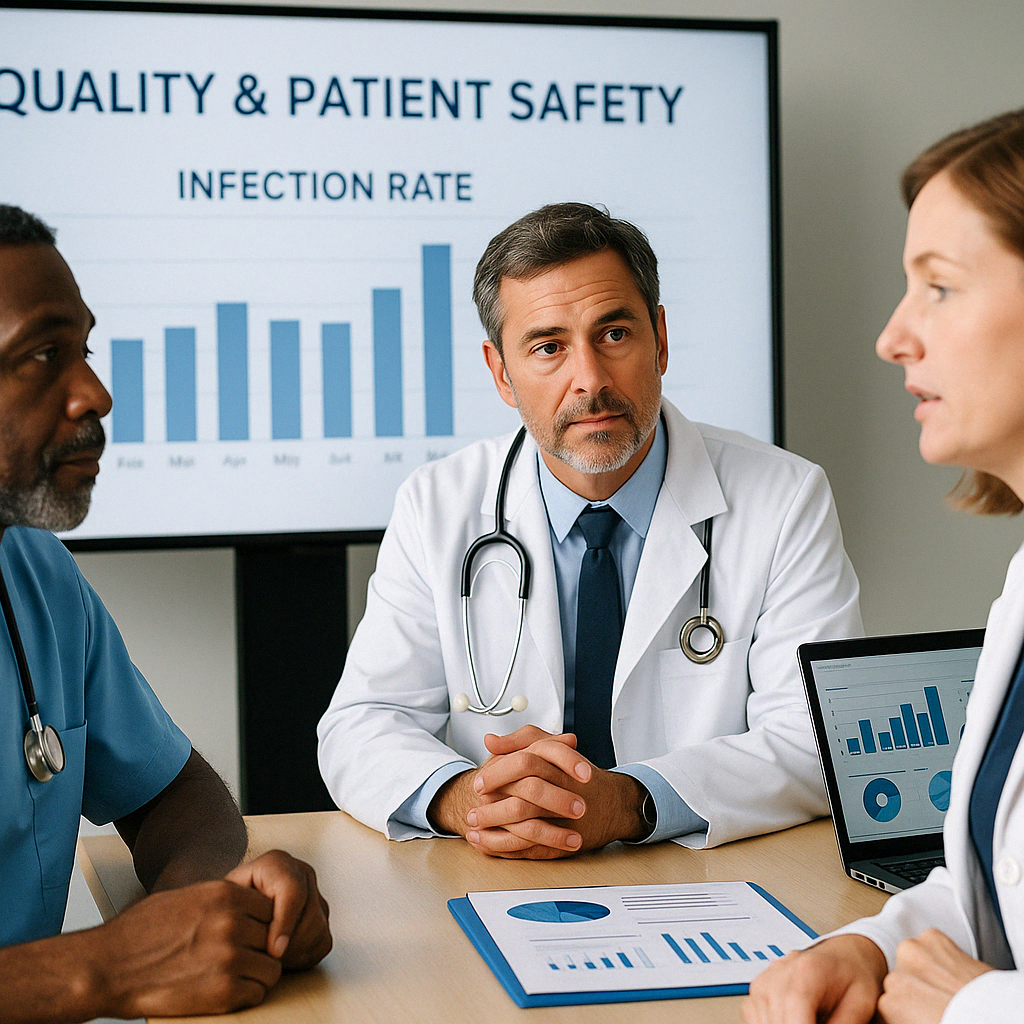
Why Every Hospital Needs a Quality and Patient Safety Program
Every hospital needs a quality and patient safety program to reduce harm, improve care, and foster a culture of accountability.
.png)
.png)
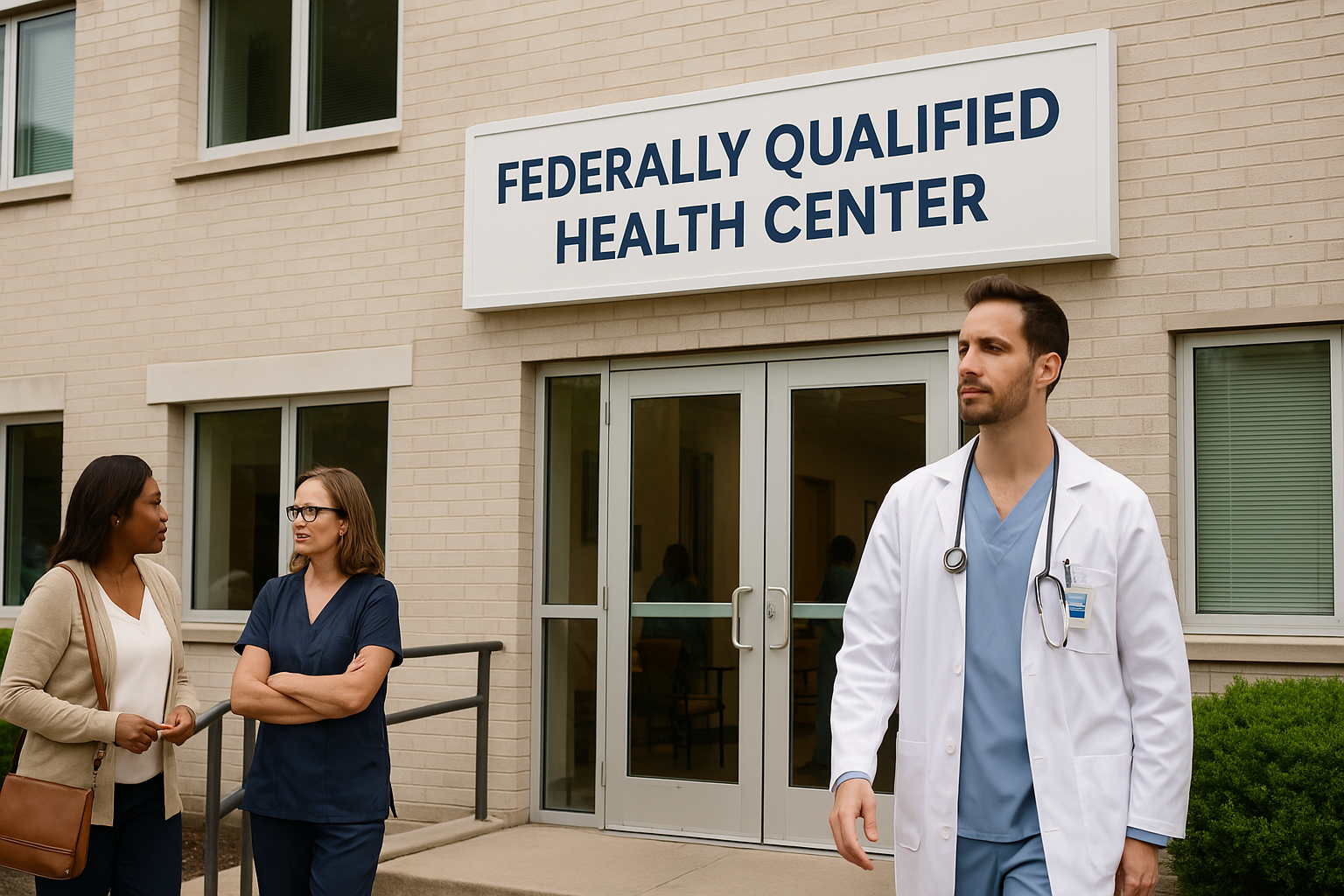
HRSA FQHC Requirements: A Comprehensive Guide for Healthcare Providers
When it comes to federally qualified health center requirements, there’s no shortage of regulations, expectations, and—depending on your perspective—opportunities.
.png)
.png)

Unlocking Funding: A Guide to Health Resources and Services Administration (HRSA) Grants
Use HRSA grants to fund external peer review programs that enhance care quality, reduce bias, and support compliance in health centers.
.png)
.png)